MalPurifier. Detoxifying Your Android, One Malicious Byte at a Time
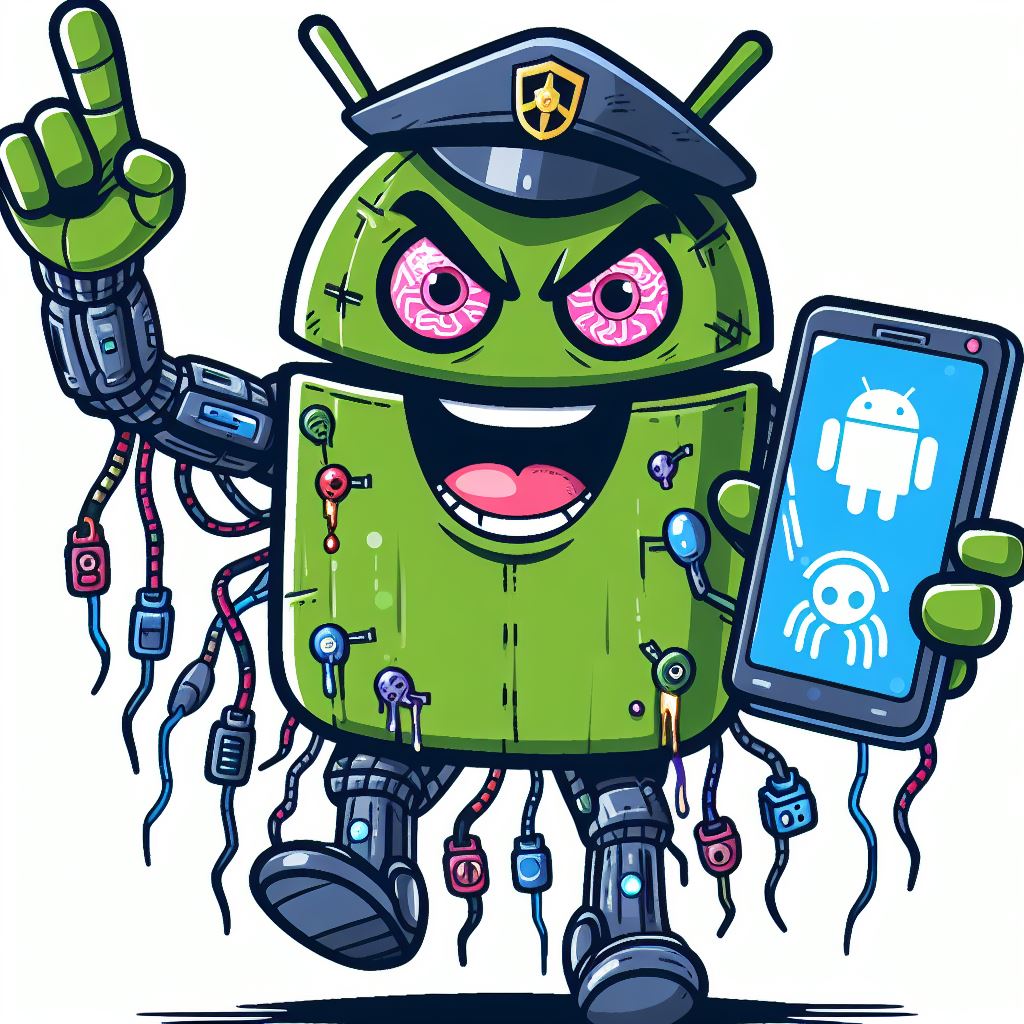
Another document to analyze. This time, it’s the riveting «MalPurifier: Enhancing Android Malware Detection with Adversarial Purification against Evasion Attacks.» Because, you know, the world really needed another paper on Android malware detection.
First, we’ll dive into the Introduction and Motivation to understand why yet another solution to the ever-escalating threats of Android malware is necessary. Spoiler alert: it’s because current machine learning-based approaches are as vulnerable as a house of cards in a windstorm.
We’ll then move on to the Experimental Setup and Results. This section will reveal how MalPurifier outperforms other defenses, achieving over 90,91% accuracy. Impressive, if you ignore the fact that it’s tested on datasets that may or may not reflect real-world scenarios.
The Defense Mechanisms section will discuss the various strategies employed by MalPurifier, such as adversarial purification and adversarial training. Because nothing says «robust defense» like throwing more adversarial examples at the problem.
Of course, no paper is complete without acknowledging its Limitations and Future Work. Here, the authors will humbly admit that their solution isn’t perfect and suggest areas for future research. Because, naturally, the quest for the perfect malware detection system is never-ending.
This analysis will provide a high-quality summary of the document, highlighting its contributions and implications for security professionals and other specialists in various fields. It will be particularly useful for those who enjoy reading about the latest and greatest in malware detection, even if the practical applications are still up for debate.
----
This document provides a comprehensive analysis of the paper titled «MalPurifier: Enhancing Android Malware Detection with Adversarial Purification against Evasion Attacks.» The analysis delves into various aspects of the paper, including the motivation behind the research, the methodology employed, the experimental setup, and the results obtained.
This analysis provides a high-quality summary of the document, offering valuable insights for security professionals, researchers, and practitioners in various fields. By understanding the strengths and limitations of the MalPurifier framework, stakeholders can better appreciate its potential applications and contributions to enhancing Android malware detection systems. The analysis is useful for those involved in cybersecurity, machine learning, and mobile application security, as it highlights innovative approaches to mitigating the risks posed by adversarial evasion attacks.
The paper titled «MalPurifier: Enhancing Android Malware Detection with Adversarial Purification against Evasion Attacks» presents a novel approach to improving the detection of Android malware, particularly in the face of adversarial evasion attacks. The paper highlights that this is the first attempt to use adversarial purification to mitigate evasion attacks in the Android ecosystem, providing a promising solution to enhance the security of Android malware detection systems.
Motivation:
📌 Prevalence of Android Malware: The paper highlights the widespread issue of Android malware, which poses significant security threats to users and devices.
📌 Evasion Techniques: Attackers often use evasion techniques to modify malware, making it difficult for traditional detection systems to identify them.
Challenges:
📌 Adversarial Attacks: it discusses the challenge posed by adversarial attacks, where small perturbations are added to malware samples to evade detection.
📌 Detection System Vulnerabilities: Existing malware detection systems are vulnerable to these adversarial attacks, leading to a need for more robust solutions.
Objective and proposed Solution:
📌 Enhancing Detection Robustness: The primary objective of the research is to enhance the robustness of Android malware detection systems against adversarial evasion attacks.
📌 Adversarial Purification: The proposed solution, MalPurifier, aims to purify adversarial examples, removing the perturbations and restoring the malware to a detectable form.
📌 Techniques Used: The system employs techniques such as autoencoders and generative adversarial networks (GANs) for the purification process.
Techniques Used in Evasion Attacks:
📌 Adversarial Examples: Attackers create adversarial examples by adding small perturbations to malware samples. These perturbations are designed to exploit vulnerabilities in the detection model’s decision boundaries.
📌 Obfuscation: Techniques such as code encryption, packing, and polymorphism are used to alter the appearance of the malware without changing its functionality.
📌 Feature Manipulation: Modifying features used by the detection model, such as adding benign features or obfuscating malicious ones, to evade detection.
Significance:
📌 Improved Security: By enhancing the detection capabilities of malware detection systems, MalPurifier aims to provide better security for Android devices.
📌 Research Contribution: The paper contributes to the field by addressing the gap in robust malware detection solutions that can withstand adversarial attacks.
Benefits
📌 High Accuracy: MalPurifier demonstrates high effectiveness, achieving accuracies over 90,91% against 37 different evasion attacks. This indicates a robust performance in detecting adversarially perturbed malware samples.
📌 Scalability: The method is easily scalable to different detection models, offering flexibility and robustness in its implementation without requiring significant modifications.
📌 Lightweight and Flexible: The use of a plug-and-play Denoising AutoEncoder (DAE) model allows for a lightweight and flexible approach to purifying adversarial malware. This ensures that the method can be integrated into existing systems with minimal overhead.
📌 Comprehensive Defense: By focusing on adversarial purification, MalPurifier addresses a critical vulnerability in ML-based malware detection systems, enhancing their overall security and robustness against sophisticated evasion techniques.
Limitations
📌 Generalization to Other Platforms: The current implementation and evaluation are focused solely on the Android ecosystem. The effectiveness of MalPurifier on other platforms, such as iOS or Windows, remains untested and uncertain.
📌 Scalability Concerns: While the paper claims scalability, the actual performance and efficiency of MalPurifier in large-scale, real-time detection scenarios have not been thoroughly evaluated. This raises questions about its practical applicability in high-volume environments.
📌 Computational Overhead: The purification process introduces additional computational overhead. Although described as lightweight, the impact on system performance, especially in resource-constrained environments, needs further investigation.
📌 Adversarial Adaptation: Attackers may develop new strategies to adapt to the purification process, potentially circumventing the defenses provided by MalPurifier. Continuous adaptation and improvement of the purification techniques are necessary to stay ahead of evolving threats.
📌 Evaluation Metrics: The evaluation primarily focuses on detection accuracy and robustness against evasion attacks. Other important metrics, such as energy consumption, user experience, and long-term efficacy, are not addressed, limiting the comprehensiveness of the assessment.
📌 Integration with Existing Systems: The paper does not extensively discuss the integration of MalPurifier with existing malware detection systems and the potential impact on their performance. Seamless integration strategies and combined performance evaluations are needed
Impact on Technology
📌 Advancement in Malware Detection: MalPurifier represents a significant technological advancement in the field of malware detection. By leveraging adversarial purification techniques, it enhances the robustness of Android malware detection systems against evasion attacks. This innovation can lead to the development of more secure and reliable malware detection tools.
📌 Adversarial Defense Mechanisms: The paper contributes to the broader field of adversarial machine learning by demonstrating the effectiveness of adversarial purification. This technique can be adapted and applied to other areas of cybersecurity, such as network intrusion detection and endpoint security, thereby improving the overall resilience of these systems against sophisticated attacks.
📌 Machine Learning Applications: The use of Denoising AutoEncoders (DAEs) and Generative Adversarial Networks (GANs) in MalPurifier showcases the potential of advanced machine learning models in cybersecurity applications. This can inspire further research and development in applying these models to other security challenges, such as phishing detection and fraud prevention.
Impact on Industry
📌 Enhanced Security for Mobile Devices: Industries that rely heavily on mobile devices, such as healthcare, finance, and retail, can benefit from the enhanced security provided by MalPurifier. By improving the detection of Android malware, these industries can better protect sensitive data and maintain the integrity of their mobile applications.
📌 Reduction in Cybersecurity Incidents: The implementation of robust malware detection systems like MalPurifier can lead to a reduction in cybersecurity incidents, such as data breaches and ransomware attacks. This can result in significant cost savings for businesses and reduce the potential for reputational damage.
📌 Compliance and Regulatory Benefits: Enhanced malware detection capabilities can help organizations comply with regulatory requirements related to data protection and cybersecurity. For example, industries subject to regulations like GDPR or HIPAA can leverage MalPurifier to ensure they meet stringent security standards.
📌 Innovation in Cybersecurity Products: Cybersecurity companies can incorporate the techniques presented in the paper into their products, leading to the development of next-generation security solutions. This can provide a competitive edge in the market and drive innovation in the cybersecurity industry.
📌 Cross-Industry Applications: While the paper focuses on Android malware detection, the underlying principles of adversarial purification can be applied across various industries. Sectors such as manufacturing, public administration, and transportation, which are also affected by malware, can adapt these techniques to enhance their cybersecurity measures.