Monthly Digest. 2024 / 07. Announcement
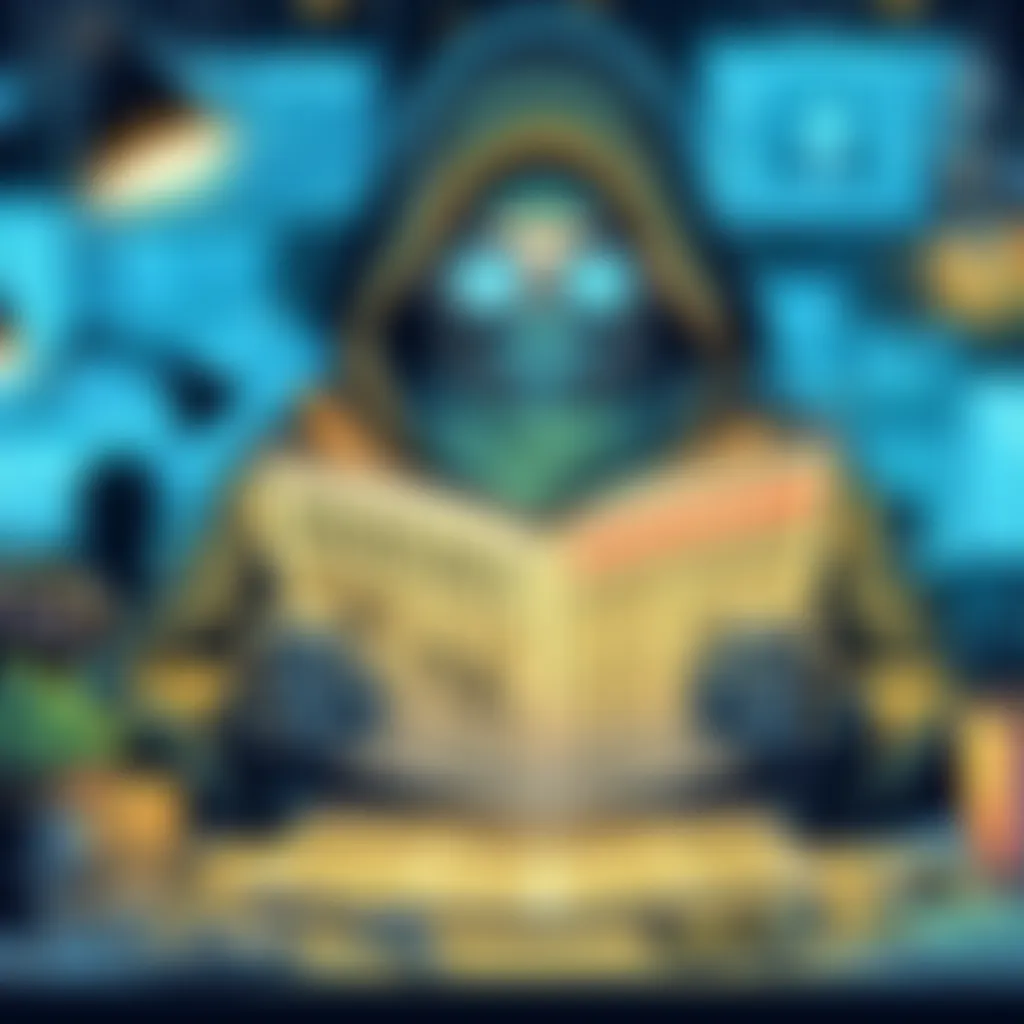
Welcome to the next edition of our Monthly Digest, your one-stop resource for staying informed on the most recent developments, insights, and best practices in the ever-evolving field of security. In this issue, we have curated a diverse collection of articles, news, and research findings tailored to both professionals and casual enthusiasts. Our digest aims to make our content is both engaging and accessible. Happy reading
Content keypoints
A. Bias in AI. Because Even Robots Can Be Sexist
Cybersecurity has traditionally been viewed through a technical lens, focusing on protecting systems and networks from external threats. However, this approach often neglects the human element, particularly the differentiated impacts of cyber threats on various gender groups. Different individuals frequently experience unique cyber threats such as online harassment, doxing, and technology-enabled abuse, which are often downplayed or omitted in conventional threat models.
Recent research and policy discussions have begun to recognize the importance of incorporating gender perspectives into cybersecurity. For instance, the UN Open-Ended Working Group (OEWG) on ICTs has highlighted the need for gender mainstreaming in cyber norm implementation and gender-sensitive capacity building. Similarly, frameworks developed by organizations like the Association for Progressive Communications (APC) provide guidelines for creating gender-responsive cybersecurity policies.
Human-centric security prioritizes understanding and addressing human behavior within the context of cybersecurity. By focusing on the psychological and interactional aspects of security, human-centric models aim to build a security culture that empowers individuals, reduces human errors, and mitigates cyber risks effectively.
SUCCESSFUL CASE STUDIES OF GENDER-BASED THREAT MODELS IN ACTION
📌 Online Harassment Detection: A social media platform implemented an AI-based system to detect and mitigate online harassment. According to UNIDIR the system used NLP techniques to analyze text for abusive language and sentiment analysis to identify harassment. The platform reported a significant reduction in harassment incidents and improved user satisfaction.
📌 Doxing Prevention: A cybersecurity firm developed a model to detect doxing attempts by analyzing patterns in data access and sharing. According to UNIDIR the model used supervised learning to classify potential doxing incidents and alert users. The firm reported a 57% increase in the detection of doxing attempts and a 32% reduction in successful doxing incidents.
📌 Gender-Sensitive Phishing Detection: A financial institution implemented a phishing detection system that included gender-specific phishing tactics. According to UNIDIR the system used transformer-based models like BERT to analyze email content for gender-specific language and emotional manipulation and reported a 22% reduction in phishing click-through rates and a 38% increase in user reporting of phishing attempts.
IMPACT OF GENDERED ASSUMPTIONS IN ALGORITHMS ON CYBERSECURITY
📌 Behavioral Differences: Studies have shown significant differences in cybersecurity behaviors between men and women. Women are often more cautious and may adopt different security practices compared to men.
📌 Perceptions and Responses: Women and men perceive and respond to cybersecurity threats differently. Women may prioritize different aspects of security, such as privacy and protection from harassment, while men may focus more on technical defenses.
📌 Gender-Disaggregated Data: Collecting and analyzing gender-disaggregated data is crucial for understanding the different impacts of cyber threats on various gender groups. This data can inform more effective and inclusive cybersecurity policies.
📌 Promoting Gender Diversity: Increasing the representation of women in cybersecurity roles can enhance the field’s overall effectiveness. Diverse teams bring varied perspectives and are better equipped to address a wide range of cyber threats.
📌 Reinforcement of Gender Stereotypes: Algorithms trained on biased datasets can reinforce existing gender stereotypes. For example, machine learning models used in cybersecurity may inherit biases from the data they are trained on, leading to gendered assumptions in threat detection and response mechanisms.
📌 Gendered Outcomes of Cyber Threats: Traditional threats, such as denial of service attacks, can have gendered outcomes like additional security burdens and targeted attacks, which are often overlooked in gender-neutral threat models.
📌 Bias in Threat Detection and Response: Automated threat detection systems, such as email filters and phishing simulations, may incorporate gendered assumptions. For example, phishing simulations often involve gender stereotyping, which can affect the accuracy and effectiveness of these security measures.
B. Security Maturity Model. Even Cybersecurity Needs to Grow Up
This document provides an analysis of the Essential Eight Maturity Model, a strategic framework developed by the Australian Cyber Security Centre to enhance cybersecurity defenses within organizations. The analysis will cover various aspects of the model, including its structure, implementation challenges, and the benefits of achieving different maturity levels.
The analysis offers valuable insights into its application and effectiveness. This analysis is particularly useful for security professionals, IT managers, and decision-makers across various industries, helping them to understand how to better protect their organizations from cyber threats and enhance their cybersecurity measures.
The Essential Eight Maturity Model provides detailed guidance and information for businesses and government entities on implementing and assessing cybersecurity practices.
📌 Purpose and Audience: designed to assist small and medium businesses, large organizations, and government entities in enhancing their cybersecurity posture. It serves as a resource to understand and apply the Essential Eight strategies effectively.
📌 Content Updates: was first published on July 16, 2021, and has been regularly updated, with the latest update on April 23, 2024. This ensures that the information remains relevant and reflects the latest cybersecurity practices and threats.
📌 Resource Availability: available as a downloadable, titled «PROTECT — Essential Eight Maturity Model, » making it accessible for offline use and easy distribution within organizations.
📌 Feedback Mechanism: users are encouraged to provide feedback on the usefulness of the information, which indicates an ongoing effort to improve the resource based on user input.
📌 Additional Services: page http://cyber.gov.au also offers links to report cyber security incidents, especially for critical infrastructure, and to sign up for alerts on new threats, highlighting a proactive approach to cybersecurity.
The Essential Eight Maturity Model FAQ provides comprehensive guidance on implementing and understanding the Essential Eight strategies. It emphasizes a proactive, risk-based approach to cybersecurity, reflecting the evolving nature of cyber threats and the importance of maintaining a balanced and comprehensive cybersecurity posture
Updates to the Essential Eight Maturity Model
📌 Reason for Updates: The Australian Signals Directorate (ASD) updates the E8MM to ensure the advice remains contemporary, fit for purpose, and practical. Updates are based on evolving malicious tradecraft, cyber threat intelligence, and feedback from Essential Eight assessment and uplift activities.
📌 Recent Updates: Recent updates include recommendations for using an automated method of asset discovery at least fortnightly and ensuring vulnerability scanners use an up-to-date vulnerability database.
Maturity Model Updates and Implementation
📌 Redefinition of Maturity Levels: The July 2021 update redefined the number of maturity levels and moved to a stronger risk-based approach to implementation. It also reintroduced Maturity Level Zero to provide a broader range of maturity level ratings.
📌 Risk-Based Approach: The model now emphasizes a risk-based approach, where circumstances like legacy systems and technical debt are considered. Choosing not to implement entire mitigation strategies where technically feasible is generally considered Maturity Level Zero.
📌 Implementation as a Package: Organizations are advised to achieve a consistent maturity level across all eight mitigation strategies before moving to a higher maturity level. This approach aims to provide a more secure baseline than achieving higher maturity levels in a few strategies to the detriment of others.
Specific Strategy Updates
📌 Application Control Changes: Additional executable content types were introduced for all maturity levels, and Maturity Level One was updated to focus on using file system access permissions to prevent malware execution
C. Human Factors in Biocybersecurity Wargames & Gamification
The paper «Human Factors in Biocybersecurity Wargames» emphasizes the need to understand vulnerabilities in the processing of biologics and how they intersect with cyber and cyber-physical systems. This understanding is crucial for ensuring product and brand integrity and protecting those served by these systems. It discusses the growing prominence of biocybersecurity and its importance to bioprocessing in both domestic and international contexts.
Scope of Bioprocessing:
📌 Bioprocessing encompasses the entire lifecycle of biosystems and their components, from initial research to development, manufacturing, and commercialization.
📌 It significantly contributes to the global economy, with applications in food, fuel, cosmetics, drugs, and green technology.
Vulnerability of Bioprocessing Pipelines:
📌 The bioprocessing pipeline is susceptible to attacks at various stages, especially where bioprocessing equipment interfaces with the internet.
📌 This vulnerability necessitates enhanced scrutiny in the design and monitoring of bioprocessing pipelines to prevent potential disruptions.
Role of Information Technology (IT):
📌 Progress in bioprocessing is increasingly dependent on automation and advanced algorithmic processes, which require substantial IT engagement.
📌 IT spending is substantial and growing, paralleling the growth in bioprocessing.
Open-Source Methodologies and Digital Growth:
📌 The adoption of open-source methodologies has led to significant growth in communication and digital technology development worldwide.
📌 This growth is further accelerated by advancements in biological computing and storage technologies.
Need for New Expertise:
📌 The integration of biocomputing, bioprocessing, and storage technologies will necessitate new expertise in both operation and defense.
📌 Basic data and process protection measures remain crucial despite technological advancements.
Importance of Wargames:
📌 To manage and secure connected bioprocessing infrastructure, IT teams must employ wargames to simulate and address potential risks.
📌 Simulations are essential for preparing organizations to handle vulnerabilities in their bioprocessing pipelines.
D. Oops, We Did It Again. CVE-2024-21111 Strikes
This document provides a comprehensive analysis of CVE-2024-21111, a critical vulnerability in Oracle VM VirtualBox affecting Windows hosts. The analysis will cover various aspects of the vulnerability, including its technical details, exploitation mechanisms, potential impacts on different industries.
This document provides a high-quality summary of the vulnerability, offering valuable insights for security professionals and other stakeholders across various industries. The analysis is beneficial for understanding the risks associated with CVE-2024-21111 and implementing effective measures to safeguard systems against potential attacks.
CVE-2024-21111 is a significant security vulnerability identified in Oracle VM VirtualBox, specifically affecting Windows hosts. This vulnerability is present in versions of VirtualBox prior to 7.0.16. It allows a low privileged attacker with logon access to the infrastructure where Oracle VM VirtualBox is executed to potentially take over the system
An attacker exploiting this vulnerability could achieve unauthorized control over the affected Oracle VM VirtualBox. The specific technical mechanism involves local privilege escalation through symbolic link following, which can lead to arbitrary file deletion and movement.
📌 Vulnerability Type: Local Privilege Escalation (LPE) allows a low privileged attacker who already has access to the system to gain higher privileges.
📌 Attack Vector and Complexity: The CVSS 3.1 vector (CVSS: 3.1/AV: L/AC: L/PR: L/UI: N/S: U/C: H/I: H/A: H) indicates that the attack vector is local (AV: L), meaning the attacker needs local access to the host. The attack complexity is low (AC: L), and no user interaction (UI: N) is required. The privileges required are low (PR: L), suggesting that an attacker with basic user privileges can exploit this vulnerability.
📌 Impact: The impacts on confidentiality, integrity, and availability are all rated high (C: H/I: H/A: H), indicating that an exploit could lead to a complete compromise of the affected system’s confidentiality, integrity, and availability.
📌 Exploitation Method: The vulnerability can be exploited through symbolic link (symlink) attacks. This involves manipulating symbolic links to redirect operations intended for legitimate files or directories to other targets, which the attacker controls. This can lead to arbitrary file deletion or movement, potentially allowing the attacker to execute arbitrary code with elevated privileges.
📌 Specific Mechanism: The vulnerability specifically involves the manipulation of log files by the VirtualBox system service (VboxSDS). The service, which runs with SYSTEM privileges, manages log files in a directory that does not have strict access controls. This allows a low privileged user to manipulate these files, potentially leading to privilege escalation. The service performs file rename/move operations recursively, and if manipulated correctly, this behavior can be abused to perform unauthorized actions.
📌 Mitigation: Users are advised to update their VirtualBox to version 7.0.16 or later, which contains the necessary patches to mitigate this vulnerability
E. When Velociraptors Meet VMs. A Forensic Fairytale
This document provides a comprehensive analysis of forensics using the Velociraptor tool. The analysis delves into various aspects of forensic investigations specific environments, which are maintaining the integrity and security of virtualized server infrastructures. Key aspects covered include data extraction methodologies, log analysis, and the identification of malicious activities within the virtual machines hosted on ESXi servers.
This analysis is particularly beneficial for security professionals, IT forensic analysts, and other specialists across different industries who are tasked with the investigation and mitigation of security breaches in virtualized environments.
This document discusses the application of Velociraptor, a forensic and incident response tool, for conducting forensic analysis on VMware ESXi environments. The use of Velociraptor in this context suggests a focus on advanced forensic techniques tailored to the complexities of virtualized server infrastructures
Key Aspects of the Analysis
📌 Data Extraction Methodologies: it discusses methods for extracting data from ESXi systems, which is vital for forensic investigations following security incidents.
📌 Log Analysis: it includes detailed procedures for examining ESXi logs, which can reveal unauthorized access or other malicious activities.
📌 Identification of Malicious Activities: by analyzing the artifacts and logs, the document outlines methods to identify and understand the nature of malicious activities that may have occurred within the virtualized environment.
📌 Use of Velociraptor for Forensics: it highlights the capabilities of Velociraptor in handling the complexities associated with ESXi systems, making it a valuable tool for forensic analysts.
Utility of the Analysis
This forensic analysis is immensely beneficial for various professionals in the cybersecurity and IT fields:
📌 Security Professionals: helps in understanding potential vulnerabilities and points of entry for security breaches within virtualized environments.
📌 Forensic Analysts: provides methodologies and tools necessary for conducting thorough investigations in environments running VMware ESXi.
📌 IT Administrators: assists in the proactive monitoring and securing of virtualized environments against potential threats.
📌 Industries Using VMware ESXi offers insights into securing and managing virtualized environments, which is crucial for maintaining the integrity and security of business operations.
F. MalPurifier. Detoxifying Your Android, One Malicious Byte at a Time
This document provides a comprehensive analysis of the paper titled «MalPurifier: Enhancing Android Malware Detection with Adversarial Purification against Evasion Attacks.» The analysis delves into various aspects of the paper, including the motivation behind the research, the methodology employed, the experimental setup, and the results obtained.
This analysis provides a high-quality summary of the document, offering valuable insights for security professionals, researchers, and practitioners in various fields. By understanding the strengths and limitations of the MalPurifier framework, stakeholders can better appreciate its potential applications and contributions to enhancing Android malware detection systems. The analysis is useful for those involved in cybersecurity, machine learning, and mobile application security, as it highlights innovative approaches to mitigating the risks posed by adversarial evasion attacks.
The paper titled «MalPurifier: Enhancing Android Malware Detection with Adversarial Purification against Evasion Attacks» presents a novel approach to improving the detection of Android malware, particularly in the face of adversarial evasion attacks. The paper highlights that this is the first attempt to use adversarial purification to mitigate evasion attacks in the Android ecosystem, providing a promising solution to enhance the security of Android malware detection systems.
Motivation:
📌 Prevalence of Android Malware: The paper highlights the widespread issue of Android malware, which poses significant security threats to users and devices.
📌 Evasion Techniques: Attackers often use evasion techniques to modify malware, making it difficult for traditional detection systems to identify them.
Challenges:
📌 Adversarial Attacks: it discusses the challenge posed by adversarial attacks, where small perturbations are added to malware samples to evade detection.
📌 Detection System Vulnerabilities: Existing malware detection systems are vulnerable to these adversarial attacks, leading to a need for more robust solutions.
Objective and proposed Solution:
📌 Enhancing Detection Robustness: The primary objective of the research is to enhance the robustness of Android malware detection systems against adversarial evasion attacks.
📌 Adversarial Purification: The proposed solution, MalPurifier, aims to purify adversarial examples, removing the perturbations and restoring the malware to a detectable form.
📌 Techniques Used: The system employs techniques such as autoencoders and generative adversarial networks (GANs) for the purification process.
Techniques Used in Evasion Attacks:
📌 Adversarial Examples: Attackers create adversarial examples by adding small perturbations to malware samples. These perturbations are designed to exploit vulnerabilities in the detection model’s decision boundaries.
📌 Obfuscation: Techniques such as code encryption, packing, and polymorphism are used to alter the appearance of the malware without changing its functionality.
📌 Feature Manipulation: Modifying features used by the detection model, such as adding benign features or obfuscating malicious ones, to evade detection.
Significance:
📌 Improved Security: By enhancing the detection capabilities of malware detection systems, MalPurifier aims to provide better security for Android devices.
Benefits
📌 High Accuracy: MalPurifier demonstrates high effectiveness, achieving accuracies over 90,91% against 37 different evasion attacks. This indicates a robust performance in detecting adversarially perturbed malware samples.
📌 Scalability: The method is easily scalable to different detection models, offering flexibility and robustness in its implementation without requiring significant modifications.
📌 Lightweight and Flexible: The use of a plug-and-play Denoising AutoEncoder (DAE) model allows for a lightweight and flexible approach to purifying adversarial malware. This ensures that the method can be integrated into existing systems with minimal overhead.
📌 Comprehensive Defense: By focusing on adversarial purification, MalPurifier addresses a critical vulnerability in ML-based malware detection systems, enhancing their overall security and robustness against sophisticated evasion techniques.
Limitations
📌 Generalization to Other Platforms: The current implementation and evaluation are focused solely on the Android ecosystem. The effectiveness of MalPurifier on other platforms, such as iOS or Windows, remains untested and uncertain.
📌 Scalability Concerns: While the paper claims scalability, the actual performance and efficiency of MalPurifier in large-scale, real-time detection scenarios have not been thoroughly evaluated. This raises questions about its practical applicability in high-volume environments.
📌 Computational Overhead: The purification process introduces additional computational overhead. Although described as lightweight, the impact on system performance, especially in resource-constrained environments, needs further investigation.
📌 Adversarial Adaptation: Attackers may develop new strategies to adapt to the purification process, potentially circumventing the defenses provided by MalPurifier. Continuous adaptation and improvement of the purification techniques are necessary to stay ahead of evolving threats.
📌 Evaluation Metrics: The evaluation primarily focuses on detection accuracy and robustness against evasion attacks. Other important metrics, such as energy consumption, user experience, and long-term efficacy, are not addressed, limiting the comprehensiveness of the assessment.
📌 Integration with Existing Systems: The paper does not extensively discuss the integration of MalPurifier with existing malware detection systems and the potential impact on their performance. Seamless integration strategies and combined performance evaluations are needed
Impact on Technology
📌 Advancement in Malware Detection: MalPurifier represents a significant technological advancement in the field of malware detection. By leveraging adversarial purification techniques, it enhances the robustness of Android malware detection systems against evasion attacks. This innovation can lead to the development of more secure and reliable malware detection tools.
📌 Adversarial Defense Mechanisms: The paper contributes to the broader field of adversarial machine learning by demonstrating the effectiveness of adversarial purification. This technique can be adapted and applied to other areas of cybersecurity, such as network intrusion detection and endpoint security, thereby improving the overall resilience of these systems against sophisticated attacks.
📌 Machine Learning Applications: The use of Denoising AutoEncoders (DAEs) and Generative Adversarial Networks (GANs) in MalPurifier showcases the potential of advanced machine learning models in cybersecurity applications. This can inspire further research and development in applying these models to other security challenges, such as phishing detection and fraud prevention.
Impact on Industry
📌 Enhanced Security for Mobile Devices: Industries that rely heavily on mobile devices, such as healthcare, finance, and retail, can benefit from the enhanced security provided by MalPurifier. By improving the detection of Android malware, these industries can better protect sensitive data and maintain the integrity of their mobile applications.
📌 Reduction in Cybersecurity Incidents: The implementation of robust malware detection systems like MalPurifier can lead to a reduction in cybersecurity incidents, such as data breaches and ransomware attacks. This can result in significant cost savings for businesses and reduce the potential for reputational damage.
📌Innovation in Cybersecurity Products: Cybersecurity companies can incorporate the techniques presented in the paper into their products, leading to the development of next-generation security solutions. This can provide a competitive edge in the market and drive innovation in the cybersecurity industry.
📌 Cross-Industry Applications: While the paper focuses on Android malware detection, the underlying principles of adversarial purification can be applied across various industries. Sectors such as manufacturing, public administration, and transportation, which are also affected by malware, can adapt these techniques to enhance their cybersecurity measures.
G. Leveraging Energy Consumption Patterns for Cyberattack Detection in IoT Systems
The proliferation of smart devices and the Internet of Things (IoT) has revolutionized various aspects of modern life, from home automation to industrial control systems. However, this technological advancement has also introduced new challenges, particularly in the realm of cybersecurity. One critical area of concern is the energy consumption of smart devices during cyberattacks, which can have far-reaching implications for device performance, longevity, and overall system resilience.
Cyberattacks on IoT devices (DDoS attacks, malware infections, botnets, ransomware, false data injection, energy consumption attacks, and cryptomining attacks) can significantly impact the energy consumption patterns of compromised devices, leading to abnormal spikes, deviations, or excessive power usage.
Monitoring and analyzing energy consumption data has emerged as a promising approach for detecting and mitigating these cyberattacks. By establishing baselines for normal energy usage patterns and employing anomaly detection techniques, deviations from expected behavior can be identified, potentially indicating the presence of malicious activities. Machine learning algorithms have demonstrated remarkable capabilities in detecting anomalies and classifying attack types based on energy consumption footprints.
The importance of addressing energy consumption during cyberattacks is multifaceted. Firstly, it enables early detection and response to potential threats, mitigating the impact of attacks and ensuring the continued functionality of critical systems. Secondly, it contributes to the overall longevity and performance of IoT devices, as excessive energy consumption can lead to overheating, reduced operational efficiency, and shortened device lifespan. Thirdly, it has economic and environmental implications, as increased energy consumption translates to higher operational costs and potentially greater carbon emissions, particularly in large-scale IoT deployments.
Furthermore, the integration of IoT devices into critical infrastructure, such as smart grids, industrial control systems, and healthcare systems, heightens the importance of addressing energy consumption during cyberattacks. Compromised devices in these environments can disrupt the balance and operation of entire systems, leading to inefficiencies, potential service disruptions, and even safety concerns.
ENERGY CONSUMPTION IMPLICATIONS
📌 Detection and Response to Cyberattacks: Monitoring the energy consumption patterns of IoT devices can serve as an effective method for detecting cyberattacks. Abnormal energy usage can indicate the presence of malicious activities, such as Distributed Denial of Service (DDoS) attacks, which can overload devices and networks, leading to increased energy consumption. By analyzing energy consumption footprints, it is possible to detect and respond to cyberattacks with high efficiency, potentially at levels of about 99,88% for detection and about 99,66% for localizing malicious software on IoT devices.
📌 Impact on Device Performance and Longevity: Cyberattacks can significantly increase the energy consumption of smart devices, which can, in turn, affect their performance and longevity. For instance, excessive energy usage can lead to overheating, reduced operational efficiency, and in the long term, can shorten the lifespan of the device. This is particularly concerning for devices that are part of critical infrastructure or those that perform essential services.
📌 Impact of Vulnerabilities: The consequences of IoT vulnerabilities are far-reaching, affecting both individual users and organizations. Cyberattacks on IoT devices can lead to privacy breaches, financial losses, and operational disruptions. For instance, the Mirai botnet attack in 2016 demonstrated the potential scale and impact of IoT-based DDoS attacks, which disrupted major online services by exploiting insecure IoT devices.
📌 Economic and Environmental Implications: The increased energy consumption of smart devices during cyberattacks has both economic and environmental implications. Economically, it can lead to higher operational costs for businesses and consumers due to increased electricity bills. Environmentally, excessive energy consumption contributes to higher carbon emissions, especially if the energy is sourced from non-renewable resources. This aspect is crucial in the context of global efforts to reduce carbon footprints and combat climate change.
📌 Energy Efficiency Challenges: Despite the benefits, smart homes face significant challenges in terms of energy efficiency. The continuous operation and connectivity of smart devices can lead to high energy consumption. To address this, IoT provides tools for better energy management, such as smart thermostats, lighting systems, and energy-efficient appliances. These tools optimize energy usage based on occupancy, weather conditions, and user preferences, significantly reducing energy waste and lowering energy bills.
📌 Challenges in Smart Grids and Energy Systems: Smart devices are increasingly integrated into smart grids and energy systems, where they play a crucial role in energy management and distribution. Cyberattacks on these devices can disrupt the balance and operation of the entire energy system, leading to inefficiencies, potential blackouts, and compromised energy security. Addressing the energy consumption of smart devices during cyberattacks is therefore vital for ensuring the stability and reliability of smart grids.
H. Hacking the Hippocratic Oath. Forensic Fun with Medical IoT
The rapid adoption of the Internet of Things (IoT) in the healthcare industry, known as the Internet of Medical Things (IoMT), has revolutionized patient care and medical operations. IoMT devices, such as wearable health monitors, implantable medical devices, and smart hospital equipment, generate and transmit vast amounts of sensitive data over networks.
Medical IoT network forensics is an emerging field that focuses on the identification, acquisition, analysis, and preservation of digital evidence from IoMT devices and networks. It plays a crucial role in investigating security incidents, data breaches, and cyber-attacks targeting healthcare organizations. The unique nature of IoMT systems, with their diverse range of devices, communication protocols, and data formats, presents significant challenges for traditional digital forensics techniques.
The primary objectives of medical IoT network forensics are:
📌 Incident Response: Rapidly respond to security incidents by identifying the source, scope, and impact of the attack, and gathering evidence to support legal proceedings or regulatory compliance.
📌 Evidence Acquisition: Develop specialized techniques to acquire and preserve digital evidence from IoMT devices, networks, and cloud-based systems while maintaining data integrity and chain of custody.
📌 Data Analysis: Analyze the collected data, including network traffic, device logs, and sensor readings, to reconstruct the events leading to the incident and identify potential vulnerabilities or attack vectors.
📌 Threat Intelligence: Leverage the insights gained from forensic investigations to enhance threat intelligence, improve security measures, and prevent future attacks on IoMT systems.
Medical IoT network forensics requires a multidisciplinary approach, combining expertise in digital forensics, cybersecurity, healthcare regulations, and IoT technologies. Forensic investigators must navigate the complexities of IoMT systems, including device heterogeneity, resource constraints, proprietary protocols, and the need to maintain patient privacy and data confidentiality.