Monthly Digest. 2024 / 06
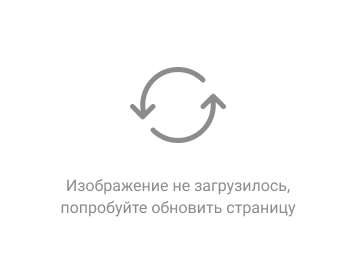
Welcome to the next edition of our Monthly Digest, your one-stop resource for staying informed on the most recent developments, insights, and best practices in the ever-evolving field of security. In this issue, we have curated a diverse collection of articles, news, and research findings tailored to both professionals and casual enthusiasts. Our digest aims to make our content is both engaging and accessible. Happy reading
Check out PDF at the end of post
A. AntiPhishStack
The paper titled «LSTM-based Stacked Generalization Model for Optimized Phishing» discusses the escalating reliance on revolutionary online web services, which has introduced heightened security risks, with persistent challenges posed by phishing attacks.
Phishing, a deceptive method through social and technical engineering, poses a severe threat to online security, aiming to obtain illicit user identities, personal account details, and bank credentials. It’s a primary concern within criminal activity, with phishers pursuing objectives such as selling stolen identities, extracting cash, exploiting vulnerabilities, or deriving financial gains.
The study aims to advance phishing detection with operating without prior phishing-specific feature knowledge. The model leverages the capabilities of Long Short-Term Memory (LSTM) networks, a type of recurrent neural network that is capable of learning order dependence in sequence prediction problems. It leverages the learning of URLs and character-level TF-IDF features symmetrically, enhancing its ability to combat emerging phishing threats.
B. NSA’s panic. AdaptTactics
The document titled «cyber actors adapt tactics for initial cloud access» released by the National Security Agency (NSA) warns of use of cyber actors have adapted their tactics to gain initial access to cloud services, as opposed to exploiting on-premise network vulnerabilities.
This shift is in response to organizations modernizing their systems and moving to cloud-based infrastructure. The high-profile cyber campaigns like the SolarWinds supply chain compromise are now expanding to sectors such as aviation, education, law enforcement, local and state councils, government financial departments, and military organizations.
The stark reality is that to breach cloud-hosted networks, these actors need only to authenticate with the cloud provider, and if they succeed, the defenses are breached. The document highlights a particularly disconcerting aspect of cloud environments: the reduced network exposure compared to on-premises systems paradoxically makes initial access a more significant linchpin.
1) Key findings
· Adaptation to Cloud Services: Cyber actors have shifted their focus from exploiting on-premises network vulnerabilities to directly targeting cloud services. This change is a response to the modernization of systems and the migration of organizational infrastructure to the cloud.
· Authentication as a Key Step: To compromise cloud-hosted networks, cyber actors must first successfully authenticate with the cloud provider. Preventing this initial access is crucial for stopping from compromising the target.
· Expansion of Targeting: Cyber actors have broadened their targeting to include sectors such as aviation, education, law enforcement, local and state councils, government financial departments, and military organizations. This expansion indicates a strategic diversification of targets for intelligence gathering.
· Use of Service and Dormant Accounts: it highlights that cyber actors have been observed using brute force attacks to access service and dormant accounts over the last 12 months. This tactic allows to gain initial access to cloud environments.
· Sophistication of cyber actors: The cyber actors can execute global supply chain compromises, such as the 2020 SolarWinds incident.
· Defense through Cybersecurity Fundamentals: The advisory emphasizes that a strong baseline of cybersecurity fundamentals can defend against cyber actors. For organizations that have transitioned to cloud infrastructure, protecting against TTPs for initial access is presented as a first line of defense.
C. NSA’s panic. Ubiquiti
Routers to Facilitate Cyber Operations» released by the Federal Bureau of Investigation (FBI), National Security Agency (NSA), US Cyber Command, and international partners warns of use of compromised Ubiquiti EdgeRouters to facilitate malicious cyber operations worldwide.
The popularity of Ubiquiti EdgeRouters is attributed to their user-friendly, Linux-based operating system, default credentials, and limited firewall protections. The routers are often shipped with insecure default configurations and do not automatically update firmware unless configured by the user.
The compromised EdgeRouters have been used by APT28 to harvest credentials, collect NTLMv2 digests, proxy network traffic, and host spear-phishing landing pages and custom tools. APT28 accessed the routers using default credentials and trojanized OpenSSH server processes. With root access to the compromised routers, the actors had unfettered access to the Linux-based operating systems to install tooling and obfuscate their identity.
APT28 also deployed custom Python scripts on the compromised routers to collect and validate stolen webmail account credentials obtained through cross-site scripting and browser-in-the-browser spear-phishing campaigns. Additionally, they exploited a critical zero-day elevation-of-privilege vulnerability in Microsoft Outlook (CVE-2023-23397) to collect NTLMv2 digests from targeted Outlook accounts and used publicly available tools to assist with NTLM relay attacks
D. NSA’s panic. SOHO
The exploitation of insecure SOHO routers by malicious cyber actors, particularly state-sponsored groups, poses a significant threat to individual users and critical infrastructure. Manufacturers are urged to adopt secure by design principles and transparency practices to mitigate these risks, while users and network defenders are advised to implement best practices for router security and remain vigilant against potential threats.
The root causes of insecure SOHO routers are multifaceted, involving both technical vulnerabilities and lapses in secure design and development practices by manufacturers, as well as negligence on the part of users in maintaining router security.
· Widespread Vulnerabilities: A significant number of vulnerabilities, totaling 226, have been identified in popular SOHO router brands. These vulnerabilities range in severity but collectively pose a substantial security risk.
· Outdated Components: Core components such as the Linux kernel and additional services like VPN in these routers are outdated. This makes them susceptible to known exploits for vulnerabilities that have long since been made public.
· Insecure Default Settings: Many routers come with easy-to-guess default passwords and use unencrypted connections. This can be easily exploited by attackers.
· Lack of Secure Design and Development: SOHO routers often lack basic security features due to insecure design and development practices. This includes the absence of automatic update capabilities and the presence of exploitable defects, particularly in web management interfaces.
· Exposure of Management Interfaces: Manufacturers frequently create devices with management interfaces exposed to the public internet by default, often without notifying the customers of this frequently unsafe configuration.
· Lack of Transparency and Accountability: There is a need for manufacturers to embrace transparency by disclosing product vulnerabilities through the CVE program and accurately classifying these vulnerabilities using the Common Weakness Enumeration (CWE) system
· Neglect of Security in Favor of Convenience and Features: Manufacturers prioritize ease of use and a wide variety of features over security, leading to routers that are «secure enough» right out of the box without considering the potential for exploitation.
· User Negligence: Many users, including IT professionals, do not follow basic security practices such as changing default passwords or updating firmware, leaving routers exposed to attacks.
· Complexity in Identifying Vulnerable Devices: Identifying specific vulnerable devices is complex due to legal and technical issues, complicating the process of mitigating these vulnerabilities.
E. Detection of Energy Consumption Cyber Attacks on Smart Devices
The paper «Detection of Energy Consumption Cyber Attacks on Smart Devices» emphasizes the rapid integration of IoT technology into smart homes, highlighting the associated security challenges due to resource constraints and unreliable networks.
· Energy Efficiency: it emphasizes the significance of energy efficiency in IoT systems, particularly in smart home environments for comfort, convenience, and security.
· Vulnerability: it discusses the vulnerability of IoT devices to cyberattacks and physical attacks due to their resource constraints. It underscores the necessity of securing these devices to ensure their effective deployment in real-world scenarios.
· Proposed Detection Framework: The authors propose a detection framework based on analyzing the energy consumption of smart devices. This framework aims to classify the attack status of monitored devices by examining their energy consumption patterns.
· Two-Stage Approach: The methodology involves a two-stage approach. The first stage uses a short time window for rough attack detection, while the second stage involves more detailed analysis.
· Lightweight Algorithm: The paper introduces a lightweight algorithm designed to detect energy consumption attacks on smart home devices. This algorithm is tailored to the limited resources of IoT devices and considers three different protocols: TCP, UDP, and MQTT.
· Packet Reception Rate Analysis: The detection technique relies on analyzing the packet reception rate of smart devices to identify abnormal behavior indicative of energy consumption attacks.
These benefits and drawbacks provide a balanced view of the proposed detection framework’s capabilities and limitations, highlighting its potential for improving smart home security.
1) Benefits
· Lightweight Detection Algorithm: The proposed algorithm is designed to be lightweight, making it suitable for resource constrained IoT devices. This ensures that the detection mechanism does not overly burden the devices it aims to protect.
· Protocol Versatility: The algorithm considers multiple communication protocols (TCP, UDP, MQTT), enhancing its applicability across various types of smart devices and network configurations.
· Two-Stage Detection Approach: The use of a two-stage detection approach (short and long-time windows) improves the accuracy of detecting energy consumption attacks while minimizing false positives. This method allows for both quick initial detection and detailed analysis.
· Real-Time Alerts: The framework promptly alerts administrators upon detecting an attack, enabling quick response and mitigation of potential threats.
· Effective Anomaly Detection: By measuring packet reception rates and analyzing energy consumption patterns, the algorithm effectively identifies deviations from normal behavior, which are indicative of cyberattacks.
2) Drawbacks
· Limited Attack Scenarios: The experimental setup has tested only specific types of attacks, which limit the generalizability of the results to other potential attack vectors not covered in the study.
· Scalability Concerns: While the algorithm is designed to be lightweight, its scalability in larger, more complex smart home environments with numerous devices and varied network conditions may require further validation.
· Dependency on Baseline Data: The effectiveness of the detection mechanism relies on accurate baseline measurements of packet reception rates and energy consumption. Any changes in the normal operating conditions of the devices could affect the baseline, potentially leading to false positives or negatives.
· Resource Constraints: Despite being lightweight, the algorithm still requires computational resources, which might be a challenge for extremely resource-limited devices. Continuous monitoring and analysis could also impact the battery life and performance of these devices.
F. MediHunt
The paper «MediHunt: A Network Forensics Framework for Medical IoT Devices» addresses the need for robust network forensics in Medical Internet of Things (MIoT) environments, particularly focusing on MQTT (Message Queuing Telemetry Transport) networks. These networks are commonly used in smart hospital environments for their lightweight communication protocol. It highlights the challenges in securing MIoT devices, which are often resource-constrained and have limited computational power. The lack of publicly available flow-based MQTT-specific datasets for training attack detection systems is mentioned as a significant challenge.
The paper presents MediHunt as an automatic network forensics solution designed for real-time detection of network flow-based traffic attacks in MQTT networks. It aims to provide a comprehensive solution for data collection, analysis, attack detection, presentation, and preservation of evidence. It is designed to detect a variety of TCP/IP layers and application layer attacks on MQTT networks. It leverages machine learning models to enhance the detection capabilities and is suitable for deployment on resource constrained MIoT devices.
Unlike many network forensics frameworks, MediHunt is specifically designed for the MIoT domain. This specialization allows it to address the unique challenges and requirements of medical IoT devices, such as resource constraints and the need for real-time attack detection.
1) Benefits
· Real-time Attack Detection: MediHunt is designed to detect network flow-based traffic attacks in real-time, which is crucial for mitigating potential damage and ensuring the security of MIoT environments.
· Comprehensive Forensic Capabilities: The framework provides a complete solution for data collection, analysis, attack detection, presentation, and preservation of evidence. This makes it a robust tool for network forensics in MIoT environments.
· Machine Learning Integration: By leveraging machine learning models, MediHunt enhances its detection capabilities. The use of a custom dataset that includes flow data for both TCP/IP layer and application layer attacks allows for more accurate and effective detection of a wide range of cyber-attacks.
· High Performance: The framework has demonstrated high performance, with F1 scores and detection accuracy exceeding 0.99 and indicates that it is highly reliable in detecting attacks on MQTT networks.
· Resource Efficiency: Despite its comprehensive capabilities, MediHunt is designed to be resource-efficient, making it suitable for deployment on resource-constrained MIoT devices like Raspberry Pi.
2) Drawbacks
· Dataset Limitations: While MediHunt uses a custom dataset for training its machine learning models, the creation and maintenance of such datasets can be challenging. The dataset needs to be regularly updated to cover new and emerging attack scenarios.
· Resource Constraints: Although MediHunt is designed to be resource-efficient, the inherent limitations of MIoT devices, such as limited computational power and memory, can still pose challenges. Ensuring that the framework runs smoothly on these devices without impacting their primary functions can be difficult.
· Complexity of Implementation: Implementing and maintaining a machine learning-based network forensics framework can be complex. It requires expertise in cybersecurity and machine learning, which may not be readily available in all healthcare settings.
· Dependence on Machine Learning Models: The effectiveness of MediHunt heavily relies on the accuracy and robustness of its machine learning models. These models need to be trained on high-quality data and regularly updated to remain effective against new types of attacks.
· Scalability Issues: While the framework is suitable for small-scale deployments on devices like Raspberry Pi, scaling it up to larger, more complex MIoT environments may present additional challenges. Ensuring consistent performance and reliability across a larger network of devices can be difficult
G. Fuxnet
The Blackjack hacking group, purportedly linked to Ukrainian intelligence services, has claimed responsibility for a cyberattack that allegedly compromised emergency detection and response capabilities in Moscow and its surrounding areas. This group has been associated with previous cyberattacks targeting internet providers and military infrastructure. Their most recent claim involves an attack on Moscollector, a company responsible for constructing and monitoring underground water, sewage, and communications infrastructure.
Regarding the infection methods, the Fuxnet malware appears to have been designed to target sensor-gateways and potentially disable them, as well as to fuzz sensors, which could lead to their malfunction or destruction.
· Unverified Claims: Team82 and Claroty have not been able to confirm the claims made by the Blackjack group regarding the impact of their cyberattack on the government’s emergency response capabilities or the extent of the damage caused by the Fuxnet malware.
· Discrepancy in Reported Impact: The Blackjack group initially claimed to have targeted 2,659 sensor-gateways, with about 1,700 being successfully attacked. However, Team82's analysis of the data leaked by Blackjack suggests that only a little more than 500 sensor gateways were actually impacted by the malware. The claim of having destroyed 87,000 sensors was also clarified by Blackjack, stating that they disabled the sensors by destroying the gateways and using M-Bus fuzzing, rather than physically destroying the sensors.
· M-Bus Fuzzing: The Blackjack group utilized a dedicated M-Bus fuzzer within the Fuxnet malware’s code to fuzz the sensors. This technique was aimed at disabling the sensors, but the exact number of sensors that were «fried» or permanently damaged as a result of this fuzzing is unknown due to the network being taken down and access to the sensor-gateways being disabled.
· Lack of Direct Evidence: Direct evidence to confirm the extent of the damage or the impact on emergency detection and response capabilities is lacking (including targeted Moscollector).
· Clarification from Blackjack: Following the publication of Team82's initial analysis, the Blackjack group reached out to provide updates and clarifications, particularly challenging the contention that only around 500 sensor-gateways had been impacted. They emphasized that the JSON files made public were only a sample of the full extent of their activity.