Bias in AI. Because Even Robots Can Be Sexist
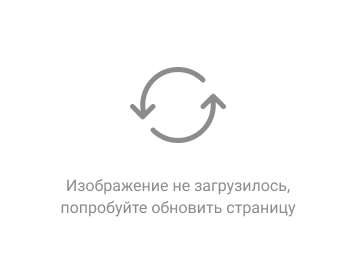
The intersection of gender and cybersecurity is an emerging field that highlights the differentiated impacts and risks faced by individuals based on their gender identities. Traditional cybersecurity models often overlook gender-specific threats such as online harassment, doxing, and technology-enabled abuse, leading to inadequate protection for vulnerable groups. This paper explores the integration of human-centric and gender-based threat models in cybersecurity, emphasizing the need for inclusive and equitable approaches. By leveraging AI and ML technologies, we can develop more effective threat detection and response systems that account for gender-specific vulnerabilities. Additionally, the paper provides a framework for developing and implementing gender-sensitive cybersecurity standards. The goal is to create a more inclusive cybersecurity environment that addresses the unique needs and experiences of all individuals, thereby enhancing overall security.
----
Cybersecurity has traditionally been viewed through a technical lens, focusing on protecting systems and networks from external threats. However, this approach often neglects the human element, particularly the differentiated impacts of cyber threats on various gender groups. Different individuals frequently experience unique cyber threats such as online harassment, doxing, and technology-enabled abuse, which are often downplayed or omitted in conventional threat models.
Recent research and policy discussions have begun to recognize the importance of incorporating gender perspectives into cybersecurity. For instance, the UN Open-Ended Working Group (OEWG) on ICTs has highlighted the need for gender mainstreaming in cyber norm implementation and gender-sensitive capacity building. Similarly, frameworks developed by organizations like the Association for Progressive Communications (APC) provide guidelines for creating gender-responsive cybersecurity policies.
Human-centric security prioritizes understanding and addressing human behavior within the context of cybersecurity. By focusing on the psychological and interactional aspects of security, human-centric models aim to build a security culture that empowers individuals, reduces human errors, and mitigates cyber risks effectively.
SUCCESSFUL CASE STUDIES OF GENDER-BASED THREAT MODELS IN ACTION
📌 Online Harassment Detection: A social media platform implemented an AI-based system to detect and mitigate online harassment. According to UNIDIR the system used NLP techniques to analyze text for abusive language and sentiment analysis to identify harassment. The platform reported a significant reduction in harassment incidents and improved user satisfaction.
📌 Doxing Prevention: A cybersecurity firm developed a model to detect doxing attempts by analyzing patterns in data access and sharing. According to UNIDIR the model used supervised learning to classify potential doxing incidents and alert users. The firm reported a 57% increase in the detection of doxing attempts and a 32% reduction in successful doxing incidents.
📌 Gender-Sensitive Phishing Detection: A financial institution implemented a phishing detection system that included gender-specific phishing tactics. According to UNIDIR the system used transformer-based models like BERT to analyze email content for gender-specific language and emotional manipulation and reported a 22% reduction in phishing click-through rates and a 38% increase in user reporting of phishing attempts.
IMPACT OF GENDERED ASSUMPTIONS IN ALGORITHMS ON CYBERSECURITY
📌 Behavioral Differences: Studies have shown significant differences in cybersecurity behaviors between men and women. Women are often more cautious and may adopt different security practices compared to men.
📌 Perceptions and Responses: Women and men perceive and respond to cybersecurity threats differently. Women may prioritize different aspects of security, such as privacy and protection from harassment, while men may focus more on technical defenses.
📌 Gender-Disaggregated Data: Collecting and analyzing gender-disaggregated data is crucial for understanding the different impacts of cyber threats on various gender groups. This data can inform more effective and inclusive cybersecurity policies.
📌 Promoting Gender Diversity: Increasing the representation of women in cybersecurity roles can enhance the field’s overall effectiveness. Diverse teams bring varied perspectives and are better equipped to address a wide range of cyber threats.
📌 Reinforcement of Gender Stereotypes: Algorithms trained on biased datasets can reinforce existing gender stereotypes. For example, machine learning models used in cybersecurity may inherit biases from the data they are trained on, leading to gendered assumptions in threat detection and response mechanisms.
📌 Misgendering and Privacy Violations: Social media platforms and other online services often use algorithms to infer user attributes, including gender. These inferences can be inaccurate, leading to misgendering and privacy violations.
📌 Gendered Outcomes of Cyber Threats: Traditional cybersecurity threats, such as denial of service attacks, can have gendered outcomes like additional security burdens and targeted attacks, which are often overlooked in gender-neutral threat models.
📌 Bias in Threat Detection and Response: Automated threat detection systems, such as email filters and phishing simulations, may incorporate gendered assumptions. For example, phishing simulations often involve gender stereotyping, which can affect the accuracy and effectiveness of these security measures.